AAAI 2024 — 3 main conference papers accepted!
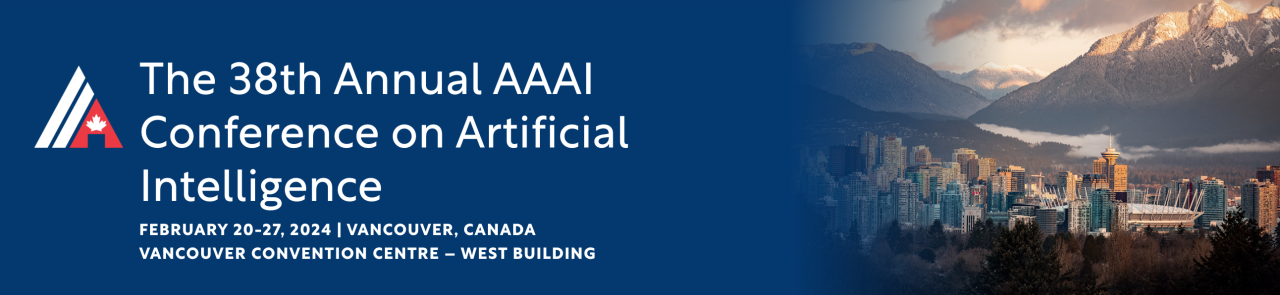
At AAAI 2024, a total of three papers from the Center for Collaborative Intelligence at Tsinghua University (TsinghuaC3I) were accepted, all of which will be presented at the main conference.
AAAI (Association for the Advance of Artificial Intelligence) is one of the top academic conferences in the field of artificial intelligence sponsored by the American Association for Artificial Intelligence. The AAAI 2024 Conference will be held from February 20 to February 27.
Paper 1
Authors:
, ,Category: Long Paper, Main Conference
Abstract: With the great success of text-conditioned diffusion models in creative text-to-image generation, various text-driven image editing approaches have attracted the attention of many researchers. However, previous works mainly focus on discreteness-sensitive instructions such as adding, removing or replacing specific objects, background elements or global styles (i.e., “hard editing”), while generally ignoring subject-binding but semantically fine-changing continuity-sensitive instructions such as actions, poses or adjectives, and so on (i.e., “soft editing”), which hampers generative AI from generating user-customized visual contents. To mitigate this predicament, we propose a spatio-temporal guided adaptive editing algorithm AdapEdit, which realizes adaptive image editing by introducing a soft-attention strategy to dynamically vary the guiding degree from the editing conditions to visual pixels from both temporal and spatial perspectives. Note our approach has a significant advantage in preserving model priors and does not require model training, fine-tuning, extra data, or optimization. We present our results over a wide variety of raw images and editing instructions, demonstrating competitive performance and showing it significantly outperforms the previous approaches.
Paper 2
Generative Multi-Modal Knowledge Retrieval with Large Language Models
Authors: Xinwei Long, Jiali Zeng, Fandong Meng, Zhiyuan Ma, Kaiyan Zhang, , Jie Zhou
Category: Long Paper, Main Conference
Abstract: Knowledge retrieval with multi-modal queries plays a crucial role in supporting knowledge-intensive multi-modal applications. However, existing methods face challenges in terms of their effectiveness and training efficiency, especially when it comes to training and integrating multiple retrievers to handle multi-modal queries. In this paper, we propose an innovative end-to-end generative framework for multi-modal knowledge retrieval. Our framework takes advantage of the fact that large language models (LLMs) can effectively serve as virtual knowledge bases, even when trained with limited data. We retrieve knowledge via a two-step process: 1) generating knowledge clues related to the queries, and 2) obtaining the relevant document by searching databases using the knowledge clue. In particular, we first introduce an object-aware prefix-tuning technique to guide multi-grained visual learning. Then, we align multi-grained visual features into the textual feature space of the LLM, employing the LLM to capture cross-modal interactions. Subsequently, we construct instruction data with a unified format for model training. Finally, we propose the knowledge-guided generation strategy to impose prior constraints in the decoding steps, thereby promoting the generation of distinctive knowledge clues. Through experiments conducted on three benchmarks, we demonstrate significant improvements ranging from 3.0% to 14.6% across all evaluation metrics when compared to strong baselines.
Paper 3
LMD: Faster Image Reconstruction with Latent Masking Diffusion
Authors: Zhiyuan Ma, Zhiyuan Yu, ,
Category: Long Paper, Main Conference
Abstract: As a class of fruitful approaches, diffusion probabilistic models (DPMs) have shown excellent advantages in high-resolution image reconstruction. On the other hand, masked autoencoders (MAEs), as popular self-supervised vision learners, have demonstrated simpler and more effective image reconstruction and transfer capabilities on downstream tasks. However, they all require extremely high training costs, either due to inherent high temporal-dependence (i.e., excessively long diffusion steps) or due to artificially low spatial-dependence (i.e., human-formulated high mask ratio, such as 0.75). To the end, this paper presents LMD, a simple but faster image reconstruction framework with Latent Masking Diffusion. First, we propose to project and reconstruct images in latent space through a pre-trained variational autoencoder, which is theoretically more efficient than in the pixel-based space. Then, we combine the advantages of MAEs and DPMs to design a progressive masking diffusion model, which gradually increases the masking proportion by three different schedulers and reconstructs the latent features from simple to difficult, without sequentially performing denoising diffusion as in DPMs or using fixed high masking ratio as in MAEs, so as to alleviate the high training time-consumption predicament. Our approach allows for learning high-capacity models and accelerate their training (by 3× or more) and barely reduces the original accuracy. Inference speed in downstream tasks also significantly outperforms the previous approaches.