ICML 2025 — 4 main conference papers accepted!
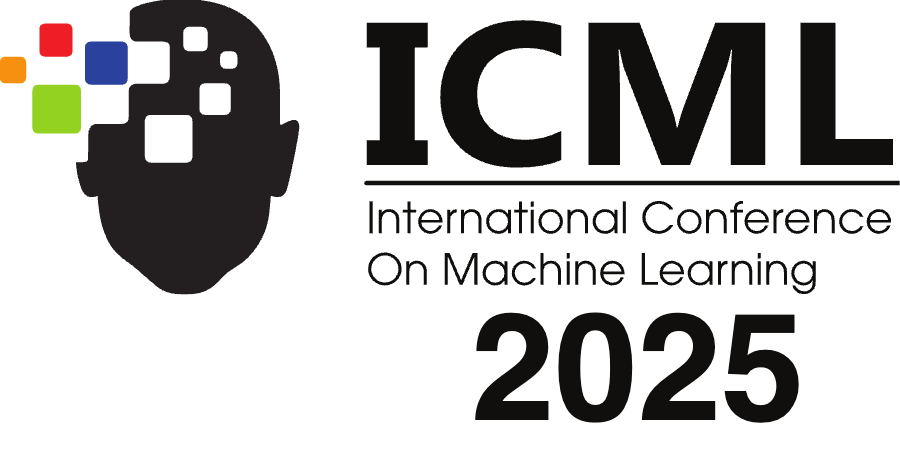
Four papers from the Center for Collaborative Intelligence at Tsinghua University (TsinghuaC3I) have been accepted to ICML 2025, all of which will be presented at the main conference.
ICML (The International Conference on Machine Learning) is globally renowned for presenting and publishing cutting-edge research on all aspects of machine learning used in closely related areas like artificial intelligence, statistics and data science, as well as important application areas such as machine vision, computational biology, speech recognition, and robotics. The ICML 2025 conference will be held from Sun. July 13th through Sat. July 19th.
Paper 1
Fourier Position Embedding: Enhancing Attention’s Periodic Extension for Length Generalization
Authors: Ermo Hua, Che Jiang, Xingtai Lv, Kaiyan Zhang, Ning Ding, Youbang Sun, Biqing Qi, Yuchen Fan, Xuekai Zhu,
Category: Long Paper, Main Conference
Abstract: Extending the context length of Language Models (LMs) by improving Rotary Position Embedding (RoPE) has become a trend. While existing works mainly address RoPE’s limitations within attention mechanism, this paper provides an analysis across nearly all parts of LMs, uncovering their adverse effects on length generalization for RoPE-based attention. Using Discrete Signal Processing theory, we show that RoPE enables periodic attention by implicitly achieving Non-Uniform Discrete Fourier Transform. However, this periodicity is undermined by the spectral damage caused by: 1) linear layers and activation functions outside of attention; 2) insufficiently trained frequency components brought by time-domain truncation. Building on our observations, we propose Fourier Position Embedding (FoPE) (Figure 1), which enhances attention’s frequency-domain properties to improve both its periodic extension and length generalization. FoPE constructs Fourier Series and zero-outs the destructive frequency components, increasing model robustness against the spectrum damage. Experiments across various model scales show that, within varying context windows, FoPE can maintain a more stable perplexity and a more consistent accuracy in a needle-in-haystack task compared to RoPE and ALiBi (Figure 2). Several analyses and ablations bring further support to our method and theoretical modeling (Figure 3).
Paper 2
Free Process Rewards Without Process Labels
Authors:
Category: Long Paper, Main Conference
Abstract: Different from its counterpart outcome reward models (ORMs), which evaluate the entire responses, a process reward model (PRM) scores a reasoning trajectory step by step, providing denser and more fine grained rewards. However, training a PRM requires labels annotated at every intermediate step, presenting significant challenges for both manual and automatic data collection. This paper aims to address this challenge. Both theoretically and empirically, we show that an \textit{implicit PRM} can be obtained at no additional cost, by simply training an ORM on the cheaper response-level labels. The only assumption is to parameterize the outcome reward as the log-likelihood ratios of the policy and reference models, which can be optimized regardless of the specific choice of loss objectives. In experiments, we instantiate our implicit PRMs with various objectives and evaluate their performance on MATH. We show that our implicit PRM outperforms a strong MCTS-based baseline \textit{á la} Math-Shepherd using less than 1/38 of the training data. Its performance can be further improved with majority voting. We further find that scaling up instructions and responses benefits our implicit PRM, and the latter brings a larger gain. Particularly, we find that our implicit PRM, when instantiated with the cross-entropy (CE) loss, is more data-efficient and can keep improving generation models even when trained with only one response per instruction, the setup that suffers from extreme data scarcity and imbalance. Further, instructions should be relevant to downstream tasks while the diversity of responses does not bring gains. Surprisingly, training on extra Math-Shepherd step labels brings no further improvements to our implicit PRM trained on only outcome data. We hope that our work will encourage a rethinking of PRM training approaches and contribute to making training PRMs more accessible.
Paper 3
How to Synthesize Text Data without Model Collapse?
Authors: Xuekai Zhu, Daixuan Cheng, Hengli Li, Kaiyan Zhang, Ermo Hua, Xingtai Lv, Ning Ding,
Category: Long Paper, Main Conference
Abstract: Model collapse in synthetic data indicates that iterative training on self-generated data leads to a gradual decline in performance. With the proliferation of AI models, synthetic data will fundamentally reshape the web data ecosystem. Future GPT- models will inevitably be trained on a blend of synthetic and human-produced data. In this paper, we focus on two questions: what is the impact of synthetic data on language model training, and how to synthesize data without model collapse? We first pre-train language models across different proportions of synthetic data, revealing a negative correlation between the proportion of synthetic data and model performance (Figure 1). We further conduct statistical analysis on synthetic data to uncover distributional shift phenomenon and over-concentration of n-gram features. Inspired by the above findings, we propose token editing on human-produced data to obtain semi-synthetic data (Figure 2). As a proof of concept, we theoretically demonstrate that token-level editing can prevent model collapse, as the test error is constrained by a finite upper bound. We conduct extensive experiments on pre-training from scratch, continual pre-training, and supervised fine-tuning (Figures 3 and 4). The results validate our theoretical proof that token-level editing improves data quality and enhances model performance.
Paper 4
MedXpertQA: Benchmarking Expert-Level Medical Reasoning and Understanding
Authors:
Category: Long Paper, Main Conference
Abstract: We introduce MedXpertQA (Figure 1), a highly challenging and comprehensive benchmark to evaluate expert-level medical knowledge and advanced reasoning. MedXpertQA includes 4,460 questions spanning 17 specialties and 11 body systems (Figure 2). It includes two subsets, Text for text evaluation and MM for multimodal evaluation. Notably, MM introduces expert-level exam questions with diverse images and rich clinical information, including patient records and examination results, setting it apart from traditional medical multimodal benchmarks with simple QA pairs generated from image captions. MedXpertQA applies rigorous filtering and augmentation to address the insufficient difficulty of existing benchmarks like MedQA, and incorporates specialty board questions to improve clinical relevance and comprehensiveness. We perform data synthesis to mitigate data leakage risk and conduct multiple rounds of expert reviews to ensure accuracy and reliability. We evaluate 16 leading models on MedXpertQA (Figures 3 and 4). Moreover, medicine is deeply connected to real-world decision-making, providing a rich and representative setting for assessing reasoning abilities beyond mathematics and code. To this end, we develop a reasoning-oriented subset to facilitate the assessment of o1-like models.