EMNLP 2023 — 3 main conference papers accepted!
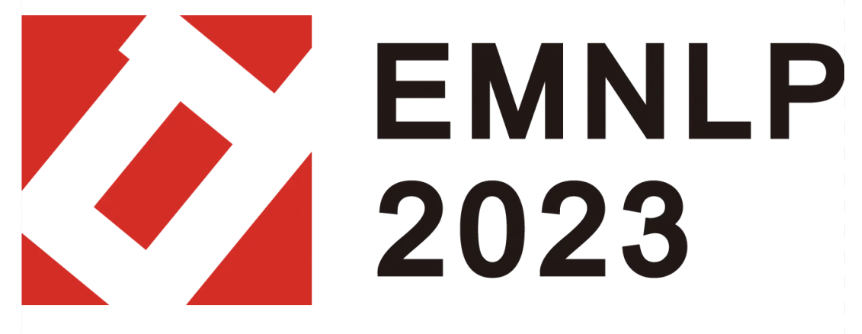
At EMNLP 2023, a total of three papers from the Center for Collaborative Intelligence at Tsinghua University (TsinghuaC3I) were accepted, all of which will be presented at the main conference.
EMNLP is a top international conference in the field of natural language processing organized by the SIGDAT group under the International Association for Computational Linguistics (ACL). EMNLP 2023 will be held offline in Singapore.
Paper 1
CRaSh: Clustering, Removing, and Sharing Enhance Fine-tuning without Full Large Language Model
Authors: Kaiyan Zhang, Ning Ding, Biqing Qi, Xuekai Zhu, Xinwei Long,
Category: Long Paper, Main Conference
Abstract: Instruction tuning has recently been recognized as an effective way of aligning Large Language Models (LLMs) to enhance their generalization ability across various tasks. However, when tuning publicly accessible, centralized LLMs with private instruction data, privacy concerns are inevitable. While direct transfer of parameterized modules between models is a plausible approach to address this, its implications and effectiveness need further exploration. This paper focuses on Offsite-Tuning (OFT), a representative technique that transfers transformer blocks between centralized LLMs and downstream emulators. Given the limited understanding of the underlying mechanism of OFT, we perform an empirical analysis on LLMs from the perspectives of representation and functional similarity. Interestingly, our findings reveal a unique modular structure within the layers of LLMs that appears to emerge as the model size expands. Simultaneously, we note subtle but potentially significant changes in representation and intermediate predictions across the layers. Inspired by these observations, we propose CRaSh, involving Clustering, Removing, and Sharing, a training-free strategy to derive improved emulators from LLMs. CRaSh significantly boosts performance of OFT with billions of parameters. Furthermore, we investigate the optimal solutions yielded by fine-tuning with and without full model through the lens of loss landscape. Our findings demonstrate a linear connectivity among these optima falling over the same basin, thereby highlighting the effectiveness of CRaSh and OFT.
Paper 2
Sparse Low-rank Adaptation of Pre-trained Language Models
Authors:
, , Qiaosen Wang, Yulin Chen, , ,Category: Long Paper, Main Conference
Abstract: Fine-tuning pre-trained large language models in a parameter-efficient manner is widely studied for its effectiveness and efficiency. The popular method of low-rank adaptation (LoRA) offers a notable approach, hypothesizing that the adaptation process is intrinsically low-dimensional. Although LoRA has demonstrated commendable performance, it is implemented with a fixed and unalterable intrinsic rank that might not always be the ideal choice. Recognizing the need for more flexible adaptation, we extend the methodology of LoRA to an innovative approach we call sparse low-rank adaptation (SoRA) that enables dynamic adjustments to the intrinsic rank during the adaptation process. We achieve this through the incorporation of a gate unit optimized with proximal gradient method in the training stage, controlling the cardinality of rank under the sparsity of the gate. In the subsequent inference stage, we eliminate the parameter blocks corresponding to the zeroed-out ranks, to reduce each SoRA module back to a concise yet rankoptimal LoRA. Our approach strengthens the representation power of LoRA by initializing it with a higher rank, while efficiently taming a temporarily increased number of parameters via updating in a sparse way. We further introduce a sparsifying scheduler for SoRA, aiming to examine the impact of the number of nonzero parameters on the model’s memorization and generalization. Our experimental results demonstrate that SoRA can outperform other baselines even with 70% retained parameters and 70% training time.
Paper 3
Enhancing Chat Language Models by Scaling High-quality Instructional Conversations
Authors: Ning Ding, Yulin Chen, Bokai Xu, Yujia Qin, Shengding Hu, , ,
Category: Long Paper, Main Conference
Abstract: Fine-tuning on instruction data has been widely validated as an effective practice for implement- ing chat language models like ChatGPT. Scal- ing the diversity and quality of such data, al- though straightforward, stands a great chance of leading to improved performance. This paper aims to improve the upper bound of open-source models further. We first provide a systematically designed, diverse, informative, large-scale dataset of instructional conversa- tions, UltraChat, which does not involve human queries. Our objective is to capture the breadth of interactions that a human might have with an AI assistant and employs a comprehensive framework to generate multi-turn conversation iteratively. UltraChat contains 1.5 million high- quality multi-turn dialogues and covers a wide range of topics and instructions. Our statis- tical analysis of UltraChat reveals its superi- ority in various key metrics, including scale, average length, diversity, coherence, etc., so- lidifying its position as a leading open-source dataset. Building upon UltraChat, we fine-tune a LLaMA model to create a powerful conver- sational model, UltraLLaMA. Our evaluations indicate that UltraLLaMA consistently outper- forms other open-source models, including Vi- cuna, the previously recognized state-of-the-art open-source model. The dataset and the model will be publicly released.